Our Automated Hyperparameter Tuning Services
As experts in hyperparameter tuning, we offer comprehensive services that enhance model accuracy, speed, and reliability across diverse machine learning tasks.
Cloud-Specific Hyperparameter Optimization
AWS SageMaker Hyperparameter Tuning: Utilize Amazon’s managed hyperparameter tuning jobs to automate and optimise critical model parameters using parallel and distributed training.
Azure Machine Learning: Automatically find the best combination of hyperparameters using Azure’s built-in AutoML capabilities, boosting model performance.
Custom Hyperparameter Optimization Strategies
We implement strategies such as grid search, random search, Bayesian optimisation, and population-based training to customise optimisation for different machine learning use cases.
Distributed and Parallel Tuning
Our methods optimise hyperparameters using distributed computing, reducing the time required for tuning. They ensure rapid convergence toward optimal configurations.
Tuning for Multiple ML Frameworks
Whether you’re using TensorFlow, PyTorch, XGBoost, or sci-kit, we tune models effectively across frameworks for cloud-specific deployments.
Model Monitoring and Retraining
Monitor model performance post-tuning and retrain periodically to adapt to changing data patterns, ensuring continued accuracy and efficiency.
Automated Pipelines for Continuous Optimization
Automate the hyperparameter tuning, minimising downtime through continuous integration and deployment (CI/CD) pipelines on AWS downtime.
Hyperparameter Tuning Consulting
Receive strategic advice on choosing the right tuning algorithms, setting optimisation budgets, and balancing performance with cost efficiency.
Unlock the potential of your visionary project with our expert team. Contact us today and let's work together to bring your dream to life.
Embark on Your Visionary Project
Why Choose Automated Hyperparameter Tuning for Your Business?
Hyperparameter tuning can drastically improve machine learning model accuracy and reduce operational overhead. Cloud-specific services like AWS and Azure offer specialised infrastructure for automated tuning.
Efficient Resource Utilization
Optimise resource consumption by using automated search techniques to identify the best parameter configurations in fewer trials.
Reduced Time to Production
Automated tuning accelerates the development cycle, allowing faster deployments and quick feedback loops.
Enhanced Model Performance
Discover the optimal combination of hyperparameters to improve prediction accuracy and overall model performance.
Cost Optimization
Benefit from cloud-specific pricing models where you pay only for the compute resources used during tuning.
Scalability
Scale hyperparameter tuning processes automatically with cloud-native features, running multiple parallel tuning jobs on demand.
Built-In Integrations
AWS and Azure provide native support for integrating data lakes, machine learning pipelines, and analytics services to create a seamless workflow
Our Clients
500+ globally customers
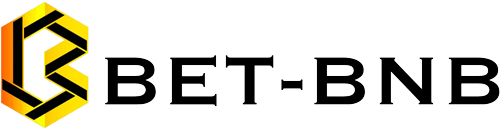
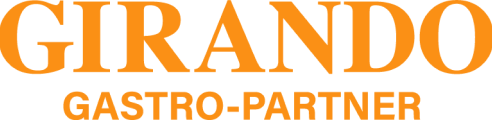
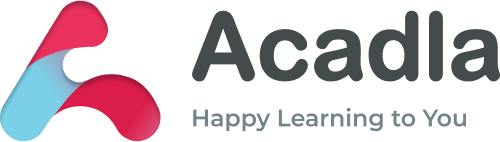

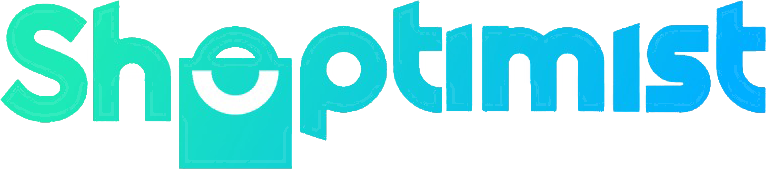
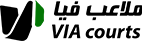

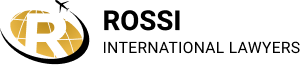
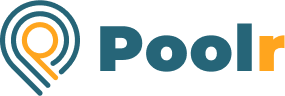
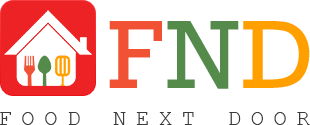
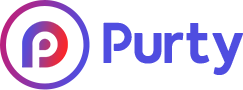
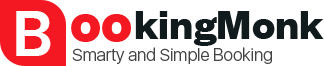
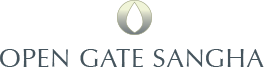
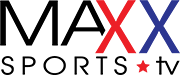
Customised Hyperparameter Tuning Solutions
At IndaPoint, we understand that different businesses require customised optimisation strategies. Our tailored hyperparameter tuning solutions on AWS and Azure help maximise model accuracy while minimising costs and training time. Whether you need tuning for deep learning models, time-series forecasting, or classification problems, we provide cloud-optimized services to deliver measurable improvements.
By focusing on these areas and using targeted keywords like "automated hyperparameter tuning," "AWS SageMaker hyperparameter optimisation," and "Azure Machine Learning AutoML," this content is designed to maximise SEO potential and attract businesses looking for cloud-optimized hyperparameter tuning services.
20+
Years Experience
50+
Talented Squad
1200+
Happy Clients
500+
Projects
Unlock the potential of your visionary project with our expert team. Contact us today and let's work together to bring your dream to life.
Embark on Your Visionary Project
Our Blogs: Feel the Beat of Innovation
Stay in sync with the latest in technology and business transformation.
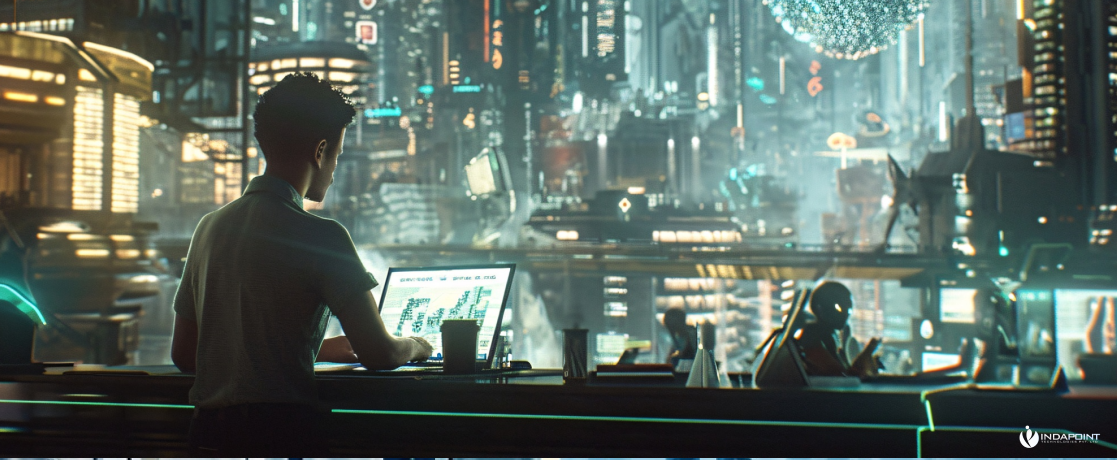
The Agentic AI Stack: Transforming Businesses with Intelligent AI Agents
The Agentic AI Stack is a powerful framework that helps businesses build intelligent AI systems that can think, learn, and collaborate like human teams. It consists of five essential layers—data retrieval, orchestration, reasoning, learning, and compliance—ensuring smooth automation, quick decision-making, and security. By using Agentic AI, companies can improve efficiency, enhance customer experiences, and drive innovation with a smart digital workforce.
March 31,2025
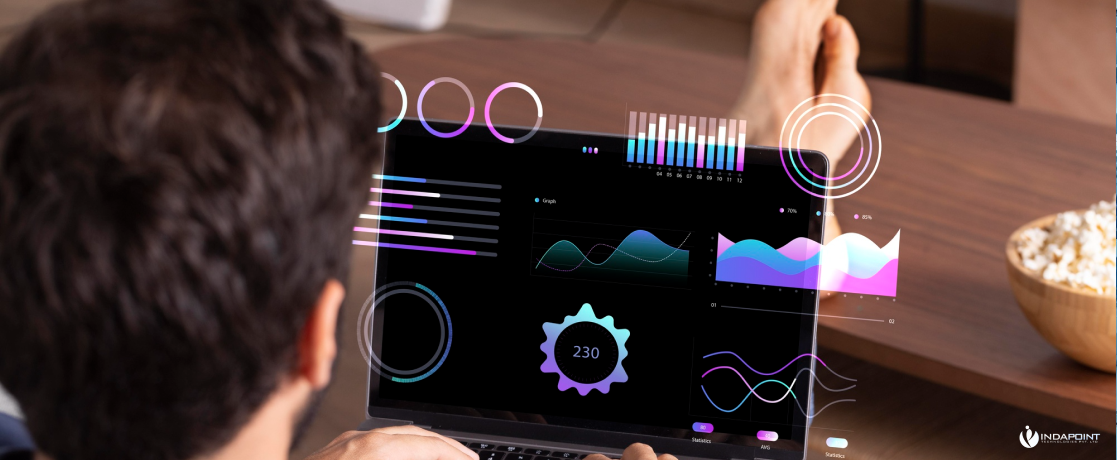
Comprehensive Guide to Data Analytics with Python
Python is a powerful programming language widely used for data analytics due to its rich ecosystem of libraries. This comprehensive guide explores various aspects of data analytics with Python, including data manipulation, visualization, statistical analysis, time series forecasting, database operations, web scraping, NLP, and machine learning.
March 21,2025
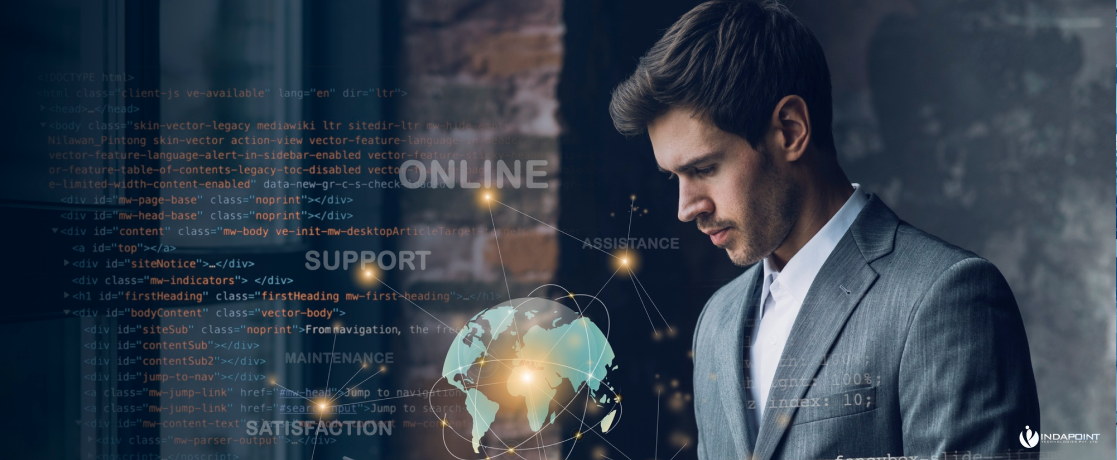
Unlocking Business Potential with Machine Learning: A Comprehensive Guide to Algorithms and Real-world Use Cases
Machine learning is transforming businesses by enabling intelligent decision-making with minimal human intervention. This guide explores essential ML algorithms, including Supervised, Unsupervised, Reinforcement Learning, Neural Networks, and Ensemble Learning. It explains how these technologies work and their real-world applications, such as customer segmentation, dynamic pricing, image recognition, fraud detection, and predictive analytics.
March 18,2025